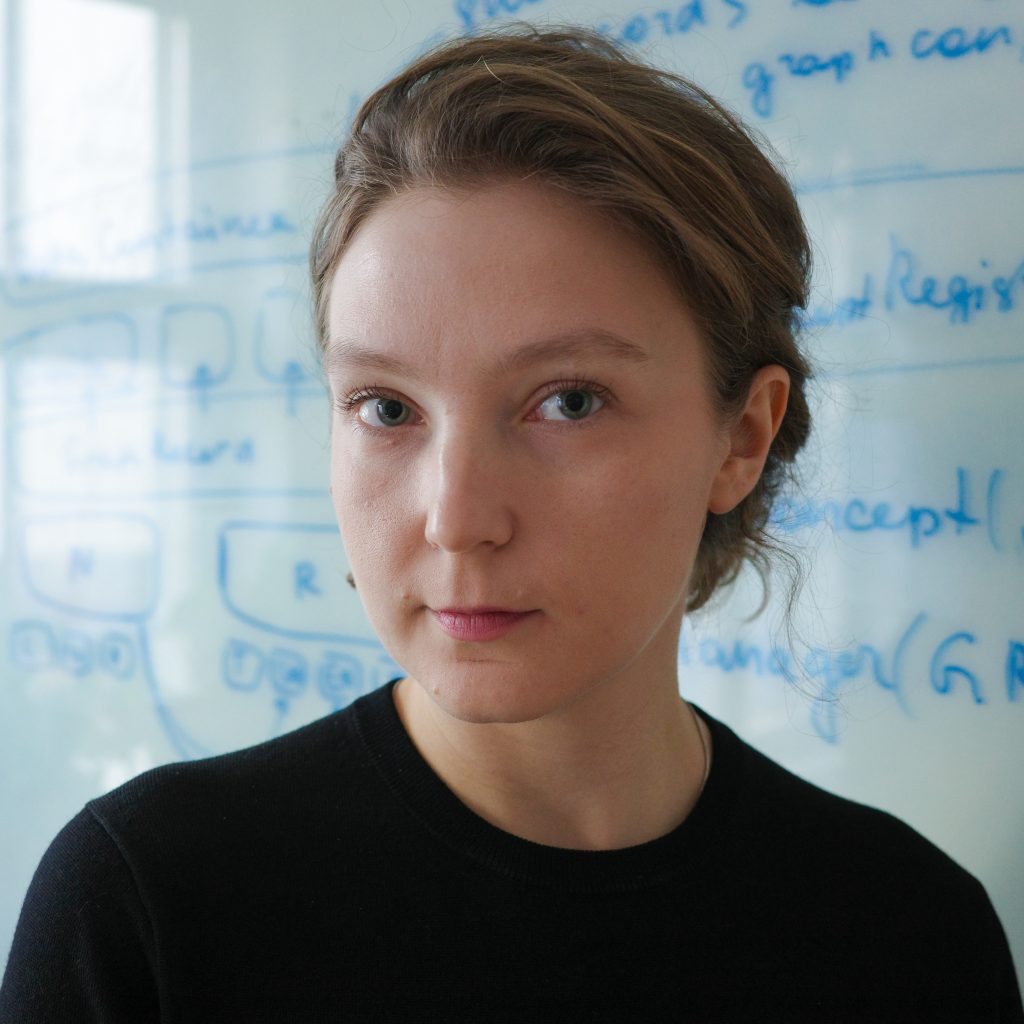
Nataliya Lopanitsyna
Syngenta, CH
Biography
Nataliya received her master's degree in Applied Mathematics and Physics from the Moscow Institute of Physics and Technology (MIPT), Moscow, and completed her PhD in 2023 at the École Polytechnique Fédérale de Lausanne (EPFL), Switzerland. Her doctoral research, at the intersection of machine learning and materials science, aimed at modeling materials under realistic conditions, using both data-driven methods and chemistry-inspired representations. Her work resulted in the development and application of machine learning models for predicting metal alloys' properties, crucial for additive manufacturing, as well as a high-throughput screening methodology for identifying candidates for novel catalysts through collaboration with industry partners.
Currently, Nataliya is working as a Postdoctoral Fellow in Cheminformatics at Syngenta. Her focus has shifted to the application of graph technology for mining synthetic routes from experimental reaction data, both from internal projects at Syngenta and external sources. By integrating these findings with predictive reaction models, her research aims to streamline and enhance the sustainability of end-to-end chemical synthesis processes.
Title
Revealing Chemical Pathways in Reaction Data through Noctis
Abstract
The Design-Make-Test-Analyze (DMTA) cycle is critical to the chemical industry, and its optimization is essential to handle challenges and ensure future readiness. Digital transformation of the cycle's steps can leverage both legacy and newly generated data to speed up new product development by reducing experimental workload. In particular, the design phase is strongly reliant on human expertise and struggle with analyzing large data bases. As a result, the development of cheminformatics tools becomes essential, enabling chemists to efficiently record, share, and analyze data while preventing oversights and eliminating duplication of work.
In this talk, I will outline the current challenges in synthesis design practices, highlighting the importance of advancing FAIR data standards and data quality in general, as well as the crucial roles that AI plays in small molecule research. I will also introduce Noctis, a Python toolkit for cheminformatics, designed to exploit chemical relations between reaction datapoints to construct knowledge graphs. Noctis offers functionality to build the reaction network combining data from different sources and an advanced suite of tools to interact with it. With the help of Noctis, one can mine synthetic routes from experimental data and combine them with reactivity model predictions to support data-driven synthesis design. This lays the foundation for our bigger ambition to use the knowledge graph as a robust base for developing a language model-driven recommendation system.