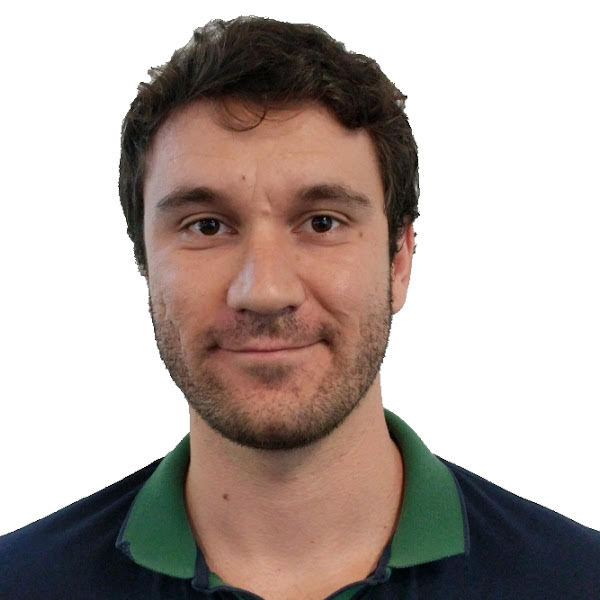
Ekin Dogus Cubuk
Google DeepMind, UK
Biography
Ekin Dogus Cubuk is a researcher at Google DeepMind where he works on deep learning and its applications to solid state physics and materials science. He received his Ph.D. from Harvard University where he studied the physics of disordered solids and battery materials using density functional theory and machine learning. After a brief postdoc at the Materials Science Department of Stanford University, he joined Google Brain in 2017. Since then, he has been studying the scaling and out-of-domain generalization properties of large neural networks, and their applications to materials discovery for applications including clean energy and information processing.
Title
Scaling up computational materials discovery via deep learning
Abstract
Deep learning models are often evaluated on validation sets sampled from the same distribution as their training sets. In the natural sciences and engineering, however, models are evaluated for their ability to generalize beyond their "training set," either in applications of discovery or theoretical modeling. This dichotomy has caused confusion in deep learning, where methods like active learning and curriculum learning do not improve performance on independent and identically distributed (IID) academic datasets such as ImageNet, while being indispensable tools in real-life applications such as autonomous driving. With the increasing interest in using machine learning in the physical sciences, this dichotomy poses an obstacle to making meaningful progress.
I will provide specific examples of this problem in the context of computational materials discovery, where graph neural networks that can predict the formation energy of inorganic crystals with unprecedented accuracy have been shown not to improve the efficiency of stable materials discovery at 0K. I will present our progress in addressing this challenge and discuss future work.