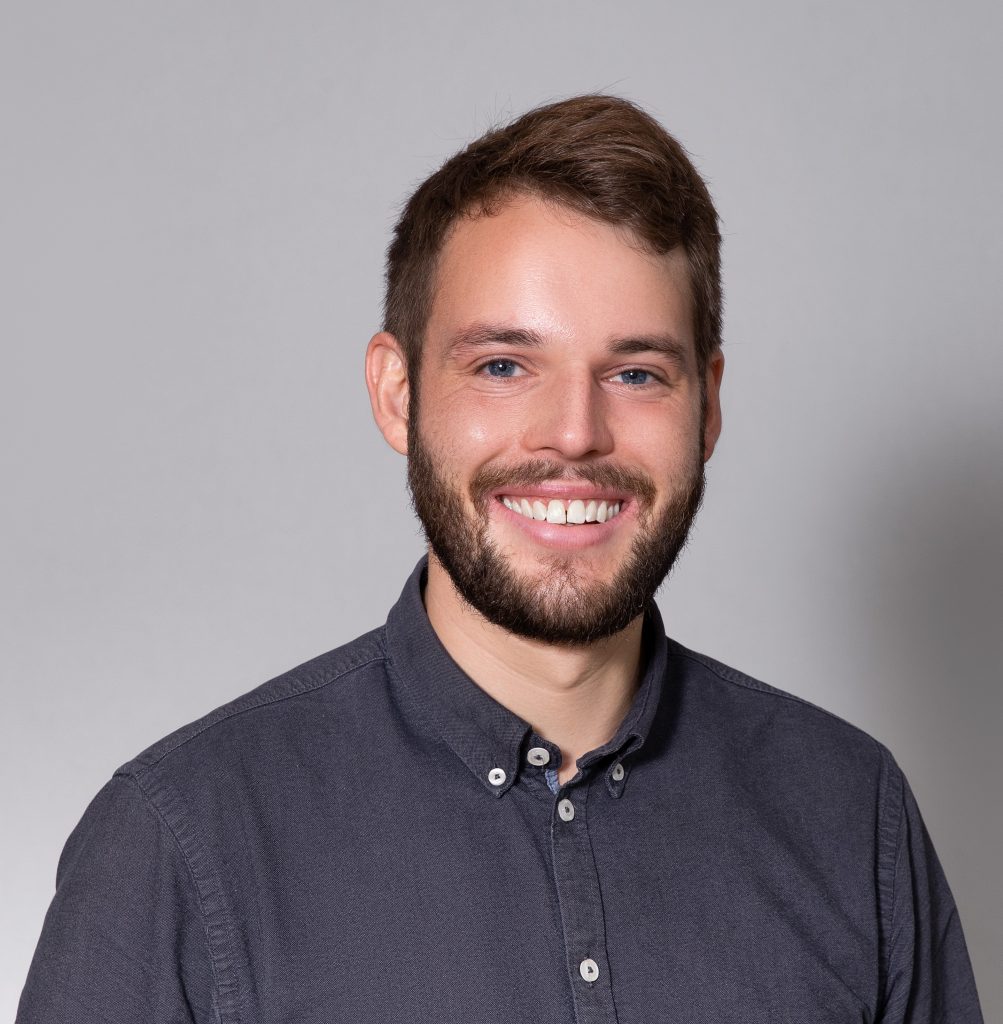
Andy Sode Anker
TU Denmark, DK
Biography
Andy S. Anker is a postdoctoral fellow at the Technical University of Denmark and a
sponsored researcher at Oxford University. He earned his PhD in 2023 from the University of
Copenhagen, under the supervision of Assoc. Prof. Kirsten Marie Ørnsbjerg Jensen. His
research focused on nanoparticles and structural analysis, employing techniques such as Total
X-ray Scattering with Pair Distribution Function analysis and Small-Angle X-ray Scattering.
Andy has developed various machine learning (ML) tools for the analysis of experimental
scattering data in materials chemistry. He was recently awarded a research fellowship of
4,000,000 DKK to develop a self-driving laboratory for the controlled synthesis of inorganic
nanomaterials, where automated analysis of scattering data via ML plays a crucial role. The
project's goal is to demonstrate how a self-driving laboratory can autonomously synthesise
nanoparticles, leveraging an ML-based data analysis feedback loop informed by scattering
measurements.
Title
Machine learning for analysis of experimental scattering data in materials chemistry
Abstract
The rapid growth of materials chemistry data, driven by advancements in large-scale radiation facilities as well as laboratory instruments, has outpaced conventional data analysis and modelling methods, which can require enormous manual effort. To address this bottleneck, supervised machine learning (ML) models are frequently trained on large datasets of physics-based simulations with the aim of being applied for experimental scattering data analysis. I will demonstrate how we have used supervised ML to identify structural models from experimental scattering data.
However, ML models trained on simulated data often struggle to perform on experimental data. I will also introduce two primary challenges in the field: 1) handling data from structures not present in the training database and 2) accounting for experimental data that contains signals not included in the simulated data.
Generative ML can be used to address both challenges by learning the underlying distribution of the data. I will discuss how we use generative ML to solve mono-metallic nanoparticles given pair distribution function data previously unseen by the model and how generative ML can be used to convert a simulated inelastic neutron scattering dataset into one that resembles an experiment, and vice versa.